diff --git a/.pre-commit-config.yaml b/.pre-commit-config.yaml
index 39e52046..39046801 100644
--- a/.pre-commit-config.yaml
+++ b/.pre-commit-config.yaml
@@ -59,17 +59,17 @@ repos:
args:
- --ignore-words-list=crate,nd,ned,strack,dota,ane,segway,fo,gool,winn,commend,bloc,nam,afterall
- - repo: https://github.com/PyCQA/docformatter
- rev: v1.7.5
- hooks:
- - id: docformatter
-
- repo: https://github.com/hadialqattan/pycln
rev: v2.4.0
hooks:
- id: pycln
args: [--all]
+# - repo: https://github.com/PyCQA/docformatter
+# rev: v1.7.5
+# hooks:
+# - id: docformatter
+
# - repo: https://github.com/asottile/yesqa
# rev: v1.4.0
# hooks:
diff --git a/README.md b/README.md
index 7a98e32a..36511313 100644
--- a/README.md
+++ b/README.md
@@ -236,7 +236,7 @@ Our key integrations with leading AI platforms extend the functionality of Ultra
-
+
@@ -246,9 +246,9 @@ Our key integrations with leading AI platforms extend the functionality of Ultra
-| Roboflow | ClearML ⭐ NEW | Comet ⭐ NEW | Neural Magic ⭐ NEW |
-| :--------------------------------------------------------------------------------------------------------------------------: | :---------------------------------------------------------------------------------------------------------------------------------: | :-------------------------------------------------------------------------------------------------------------------------------------------------------: | :----------------------------------------------------------------------------------------------------: |
-| Label and export your custom datasets directly to YOLOv8 for training with [Roboflow](https://roboflow.com/?ref=ultralytics) | Automatically track, visualize and even remotely train YOLOv8 using [ClearML](https://cutt.ly/yolov5-readme-clearml) (open-source!) | Free forever, [Comet](https://bit.ly/yolov8-readme-comet) lets you save YOLOv8 models, resume training, and interactively visualize and debug predictions | Run YOLOv8 inference up to 6x faster with [Neural Magic DeepSparse](https://bit.ly/yolov5-neuralmagic) |
+| Roboflow | ClearML ⭐ NEW | Comet ⭐ NEW | Neural Magic ⭐ NEW |
+| :--------------------------------------------------------------------------------------------------------------------------: | :-------------------------------------------------------------------------------------------------------------: | :-------------------------------------------------------------------------------------------------------------------------------------------------------: | :----------------------------------------------------------------------------------------------------: |
+| Label and export your custom datasets directly to YOLOv8 for training with [Roboflow](https://roboflow.com/?ref=ultralytics) | Automatically track, visualize and even remotely train YOLOv8 using [ClearML](https://clear.ml/) (open-source!) | Free forever, [Comet](https://bit.ly/yolov8-readme-comet) lets you save YOLOv8 models, resume training, and interactively visualize and debug predictions | Run YOLOv8 inference up to 6x faster with [Neural Magic DeepSparse](https://bit.ly/yolov5-neuralmagic) |
##
Ultralytics HUB
diff --git a/README.zh-CN.md b/README.zh-CN.md
index f89b2bdb..eec0a468 100644
--- a/README.zh-CN.md
+++ b/README.zh-CN.md
@@ -238,7 +238,7 @@ Ultralytics 提供了 YOLOv8 的交互式笔记本,涵盖训练、验证、跟
-
+
@@ -248,9 +248,9 @@ Ultralytics 提供了 YOLOv8 的交互式笔记本,涵盖训练、验证、跟
-| Roboflow | ClearML ⭐ NEW | Comet ⭐ NEW | Neural Magic ⭐ NEW |
-| :--------------------------------------------------------------------------------: | :----------------------------------------------------------------------------: | :----------------------------------------------------------------------------------: | :-----------------------------------------------------------------------------------: |
-| 使用 [Roboflow](https://roboflow.com/?ref=ultralytics) 将您的自定义数据集直接标记并导出至 YOLOv8 进行训练 | 使用 [ClearML](https://cutt.ly/yolov5-readme-clearml)(开源!)自动跟踪、可视化,甚至远程训练 YOLOv8 | 免费且永久,[Comet](https://bit.ly/yolov8-readme-comet) 让您保存 YOLOv8 模型、恢复训练,并以交互式方式查看和调试预测 | 使用 [Neural Magic DeepSparse](https://bit.ly/yolov5-neuralmagic) 使 YOLOv8 推理速度提高多达 6 倍 |
+| Roboflow | ClearML ⭐ NEW | Comet ⭐ NEW | Neural Magic ⭐ NEW |
+| :--------------------------------------------------------------------------------: | :--------------------------------------------------------: | :----------------------------------------------------------------------------------: | :-----------------------------------------------------------------------------------: |
+| 使用 [Roboflow](https://roboflow.com/?ref=ultralytics) 将您的自定义数据集直接标记并导出至 YOLOv8 进行训练 | 使用 [ClearML](https://clear.ml/)(开源!)自动跟踪、可视化,甚至远程训练 YOLOv8 | 免费且永久,[Comet](https://bit.ly/yolov8-readme-comet) 让您保存 YOLOv8 模型、恢复训练,并以交互式方式查看和调试预测 | 使用 [Neural Magic DeepSparse](https://bit.ly/yolov5-neuralmagic) 使 YOLOv8 推理速度提高多达 6 倍 |
## Ultralytics HUB
diff --git a/docs/en/datasets/explorer/api.md b/docs/en/datasets/explorer/api.md
index 2d98ffa2..2317db0e 100644
--- a/docs/en/datasets/explorer/api.md
+++ b/docs/en/datasets/explorer/api.md
@@ -8,7 +8,7 @@ keywords: Ultralytics Explorer API, Dataset Exploration, SQL Queries, Vector Sim
## Introduction
-
+
The Explorer API is a Python API for exploring your datasets. It supports filtering and searching your dataset using SQL queries, vector similarity search and semantic search.
## Installation
@@ -42,7 +42,7 @@ dataframe = explorer.get_similar(idx=0)
Embeddings table for a given dataset and model pair is only created once and reused. These use [LanceDB](https://lancedb.github.io/lancedb/) under the hood, which scales on-disk, so you can create and reuse embeddings for large datasets like COCO without running out of memory.
In case you want to force update the embeddings table, you can pass `force=True` to `create_embeddings_table` method.
-You can direclty access the LanceDB table object to perform advanced analysis. Learn more about it in [Working with table section](#4-advanced---working-with-embeddings-table)
+You can directly access the LanceDB table object to perform advanced analysis. Learn more about it in [Working with table section](#4-advanced---working-with-embeddings-table)
## 1. Similarity Search
diff --git a/docs/en/datasets/explorer/index.md b/docs/en/datasets/explorer/index.md
index 5b130c78..29e89dd0 100644
--- a/docs/en/datasets/explorer/index.md
+++ b/docs/en/datasets/explorer/index.md
@@ -10,7 +10,7 @@ keywords: Ultralytics Explorer, CV Dataset Tools, Semantic Search, SQL Dataset Q
-
+
Ultralytics Explorer is a tool for exploring CV datasets using semantic search, SQL queries, vector similarity search and even using natural language. It is also a Python API for accessing the same functionality.
### Installation of optional dependencies
diff --git a/docs/en/modes/predict.md b/docs/en/modes/predict.md
index aa19c4c4..a2bb6158 100644
--- a/docs/en/modes/predict.md
+++ b/docs/en/modes/predict.md
@@ -441,6 +441,7 @@ All Ultralytics `predict()` calls will return a list of `Results` objects:
| `masks` | `Masks, optional` | A Masks object containing the detection masks. |
| `probs` | `Probs, optional` | A Probs object containing probabilities of each class for classification task. |
| `keypoints` | `Keypoints, optional` | A Keypoints object containing detected keypoints for each object. |
+| `obb` | `OBB, optional` | A OBB object containing the oriented detection bounding boxes. |
| `speed` | `dict` | A dictionary of preprocess, inference, and postprocess speeds in milliseconds per image. |
| `names` | `dict` | A dictionary of class names. |
| `path` | `str` | The path to the image file. |
@@ -606,6 +607,44 @@ Here's a table summarizing the methods and properties for the `Probs` class:
For more details see the `Probs` class [documentation](../reference/engine/results.md#ultralytics.engine.results.Probs).
+### OBB
+
+`OBB` object can be used to index, manipulate, and convert oriented bounding boxes to different formats.
+
+!!! Example "OBB"
+
+ ```python
+ from ultralytics import YOLO
+
+ # Load a pretrained YOLOv8n model
+ model = YOLO('yolov8n-obb.pt')
+
+ # Run inference on an image
+ results = model('bus.jpg') # results list
+
+ # View results
+ for r in results:
+ print(r.obb) # print the OBB object containing the oriented detection bounding boxes
+ ```
+
+Here is a table for the `OBB` class methods and properties, including their name, type, and description:
+
+| Name | Type | Description |
+|-------------|---------------------------|-----------------------------------------------------------------------|
+| `cpu()` | Method | Move the object to CPU memory. |
+| `numpy()` | Method | Convert the object to a numpy array. |
+| `cuda()` | Method | Move the object to CUDA memory. |
+| `to()` | Method | Move the object to the specified device. |
+| `conf` | Property (`torch.Tensor`) | Return the confidence values of the boxes. |
+| `cls` | Property (`torch.Tensor`) | Return the class values of the boxes. |
+| `id` | Property (`torch.Tensor`) | Return the track IDs of the boxes (if available). |
+| `xyxy` | Property (`torch.Tensor`) | Return the horizontal boxes in xyxy format. |
+| `xywhr` | Property (`torch.Tensor`) | Return the rotated boxes in xywhr format. |
+| `xyxyxyxy` | Property (`torch.Tensor`) | Return the rotated boxes in xyxyxyxy format. |
+| `xyxyxyxyn` | Property (`torch.Tensor`) | Return the rotated boxes in xyxyxyxy format normalized by image size. |
+
+For more details see the `OBB` class [documentation](../reference/engine/results.md#ultralytics.engine.results.OBB).
+
## Plotting Results
You can use the `plot()` method of a `Result` objects to visualize predictions. It plots all prediction types (boxes, masks, keypoints, probabilities, etc.) contained in the `Results` object onto a numpy array that can then be shown or saved.
diff --git a/docs/en/reference/data/split_dota.md b/docs/en/reference/data/split_dota.md
index 1c2cf23d..ae3dfaa2 100644
--- a/docs/en/reference/data/split_dota.md
+++ b/docs/en/reference/data/split_dota.md
@@ -1,3 +1,8 @@
+---
+description: Detailed guide on using YOLO with DOTA dataset for object detection, including dataset preparation, image splitting, and label handling.
+keywords: Ultralytics, YOLO, DOTA dataset, object detection, image processing, python, dataset preparation, image splitting, label handling, YOLO with DOTA, computer vision, AI, machine learning
+---
+
# Reference for `ultralytics/data/split_dota.py`
!!! Note
diff --git a/docs/en/reference/models/yolo/obb/predict.md b/docs/en/reference/models/yolo/obb/predict.md
index 8279a641..159f59a4 100644
--- a/docs/en/reference/models/yolo/obb/predict.md
+++ b/docs/en/reference/models/yolo/obb/predict.md
@@ -1,3 +1,8 @@
+---
+description: Discover OBBPredictor for YOLO, specializing in Oriented Bounding Box predictions. Essential for advanced object detection with Ultralytics YOLO.
+keywords: Ultralytics, OBBPredictor, YOLO, Oriented Bounding Box, object detection, advanced object detection, YOLO model, deep learning, AI, machine learning, computer vision, OBB detection
+---
+
# Reference for `ultralytics/models/yolo/obb/predict.py`
!!! Note
diff --git a/docs/en/reference/models/yolo/obb/train.md b/docs/en/reference/models/yolo/obb/train.md
index 3888aff8..1a9c8ec6 100644
--- a/docs/en/reference/models/yolo/obb/train.md
+++ b/docs/en/reference/models/yolo/obb/train.md
@@ -1,3 +1,8 @@
+---
+description: Master the Ultralytics YOLO OBB Trainer: A specialized tool for training YOLO models using Oriented Bounding Boxes. Features detailed usage, model initialization, and training processes.
+keywords: Ultralytics, YOLO OBB Trainer, Oriented Bounding Box, OBB model training, YOLO model training, computer vision, deep learning, machine learning, YOLO object detection, model initialization, YOLO training process
+---
+
# Reference for `ultralytics/models/yolo/obb/train.py`
!!! Note
diff --git a/docs/en/reference/models/yolo/obb/val.md b/docs/en/reference/models/yolo/obb/val.md
index aeeccea1..b18db7ec 100644
--- a/docs/en/reference/models/yolo/obb/val.md
+++ b/docs/en/reference/models/yolo/obb/val.md
@@ -1,3 +1,8 @@
+---
+description: Learn about Ultralytics' advanced OBBValidator, an extension of YOLO object detection for oriented bounding box validation.
+keywords: Ultralytics, YOLO, OBBValidator, object detection, oriented bounding box, OBB, machine learning, AI, deep learning, Python, YOLO model, image processing, computer vision, YOLO object detection
+---
+
# Reference for `ultralytics/models/yolo/obb/val.py`
!!! Note
diff --git a/docs/en/tasks/obb.md b/docs/en/tasks/obb.md
index 48a8a26e..e4bb62b7 100644
--- a/docs/en/tasks/obb.md
+++ b/docs/en/tasks/obb.md
@@ -18,6 +18,11 @@ The output of an oriented object detector is a set of rotated bounding boxes tha
YOLOv8 OBB models use the `-obb` suffix, i.e. `yolov8n-obb.pt` and are pretrained on [DOTAv1](https://github.com/ultralytics/ultralytics/blob/main/ultralytics/cfg/datasets/DOTAv1.yaml).
+
+| Ships Detection using OBB | Vehicle Detection using OBB |
+|:-------------------------------------------------------------------------------------------------------------------------------:|:---------------------------------------------------------------------------------------------------------------------------------:|
+| 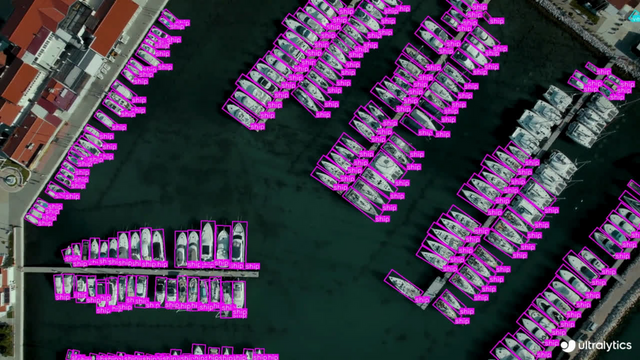 |  |
+
## [Models](https://github.com/ultralytics/ultralytics/tree/main/ultralytics/cfg/models/v8)
YOLOv8 pretrained OBB models are shown here, which are pretrained on the [DOTAv1](https://github.com/ultralytics/ultralytics/blob/main/ultralytics/cfg/datasets/DOTAv1.yaml) dataset.
diff --git a/docs/en/yolov5/tutorials/clearml_logging_integration.md b/docs/en/yolov5/tutorials/clearml_logging_integration.md
index 1945de2c..48fce1ee 100644
--- a/docs/en/yolov5/tutorials/clearml_logging_integration.md
+++ b/docs/en/yolov5/tutorials/clearml_logging_integration.md
@@ -10,7 +10,7 @@ keywords: ClearML, YOLOv5, Ultralytics, AI toolbox, training data, remote traini
## About ClearML
-[ClearML](https://cutt.ly/yolov5-tutorial-clearml) is an [open-source](https://github.com/allegroai/clearml) toolbox designed to save you time ⏱️.
+[ClearML](https://clear.ml/) is an [open-source](https://github.com/allegroai/clearml) toolbox designed to save you time ⏱️.
🔨 Track every YOLOv5 training run in the experiment manager
@@ -36,7 +36,7 @@ And so much more. It's up to you how many of these tools you want to use, you ca
To keep track of your experiments and/or data, ClearML needs to communicate to a server. You have 2 options to get one:
-Either sign up for free to the [ClearML Hosted Service](https://cutt.ly/yolov5-tutorial-clearml) or you can set up your own server, see [here](https://clear.ml/docs/latest/docs/deploying_clearml/clearml_server). Even the server is open-source, so even if you're dealing with sensitive data, you should be good to go!
+Either sign up for free to the [ClearML Hosted Service](https://clear.ml/) or you can set up your own server, see [here](https://clear.ml/docs/latest/docs/deploying_clearml/clearml_server). Even the server is open-source, so even if you're dealing with sensitive data, you should be good to go!
- Install the `clearml` python package:
diff --git a/docs/en/yolov5/tutorials/train_custom_data.md b/docs/en/yolov5/tutorials/train_custom_data.md
index 51a6027e..41f5cfc8 100644
--- a/docs/en/yolov5/tutorials/train_custom_data.md
+++ b/docs/en/yolov5/tutorials/train_custom_data.md
@@ -34,12 +34,9 @@ Creating a custom model to detect your objects is an iterative process of collec
For more details see [Ultralytics Licensing](https://ultralytics.com/license).
-### 1. Create Dataset
-
YOLOv5 models must be trained on labelled data in order to learn classes of objects in that data. There are two options for creating your dataset before you start training:
-
-Use Roboflow to create your dataset in YOLO format 🌟
+## Option 1: Create a Roboflow Dataset
### 1.1 Collect Images
@@ -68,7 +65,7 @@ Note: YOLOv5 does online augmentation during training, so we do not recommend ap
- **Auto-Orient** - to strip EXIF orientation from your images.
- **Resize (Stretch)** - to the square input size of your model (640x640 is the YOLOv5 default).
-Generating a version will give you a point in time snapshot of your dataset so you can always go back and compare your future model training runs against it, even if you add more images or change its configuration later.
+Generating a version will give you a snapshot of your dataset, so you can always go back and compare your future model training runs against it, even if you add more images or change its configuration later.

@@ -76,14 +73,9 @@ Export in `YOLOv5 Pytorch` format, then copy the snippet into your training scri

-Now continue with `2. Select a Model`.
+## Option 2: Create a Manual Dataset
-
-
-
-Or manually prepare your dataset
-
-### 1.1 Create dataset.yaml
+### 2.1 Create `dataset.yaml`
[COCO128](https://www.kaggle.com/ultralytics/coco128) is an example small tutorial dataset composed of the first 128 images in [COCO](http://cocodataset.org/#home) train2017. These same 128 images are used for both training and validation to verify our training pipeline is capable of overfitting. [data/coco128.yaml](https://github.com/ultralytics/yolov5/blob/master/data/coco128.yaml), shown below, is the dataset config file that defines 1) the dataset root directory `path` and relative paths to `train` / `val` / `test` image directories (or *.txt files with image paths) and 2) a class `names` dictionary:
@@ -105,7 +97,7 @@ names:
79: toothbrush
```
-### 1.2 Create Labels
+### 2.2 Create Labels
After using an annotation tool to label your images, export your labels to **YOLO format**, with one `*.txt` file per image (if no objects in image, no `*.txt` file is required). The `*.txt` file specifications are:
@@ -120,7 +112,7 @@ The label file corresponding to the above image contains 2 persons (class `0`) a
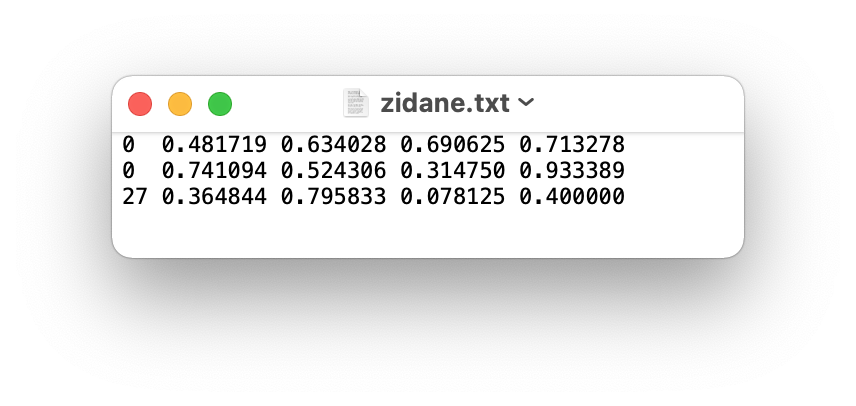
-### 1.3 Organize Directories
+### 2.3 Organize Directories
Organize your train and val images and labels according to the example below. YOLOv5 assumes `/coco128` is inside a `/datasets` directory **next to** the `/yolov5` directory. **YOLOv5 locates labels automatically for each image** by replacing the last instance of `/images/` in each image path with `/labels/`. For example:
@@ -130,15 +122,14 @@ Organize your train and val images and labels according to the example below. YO
```
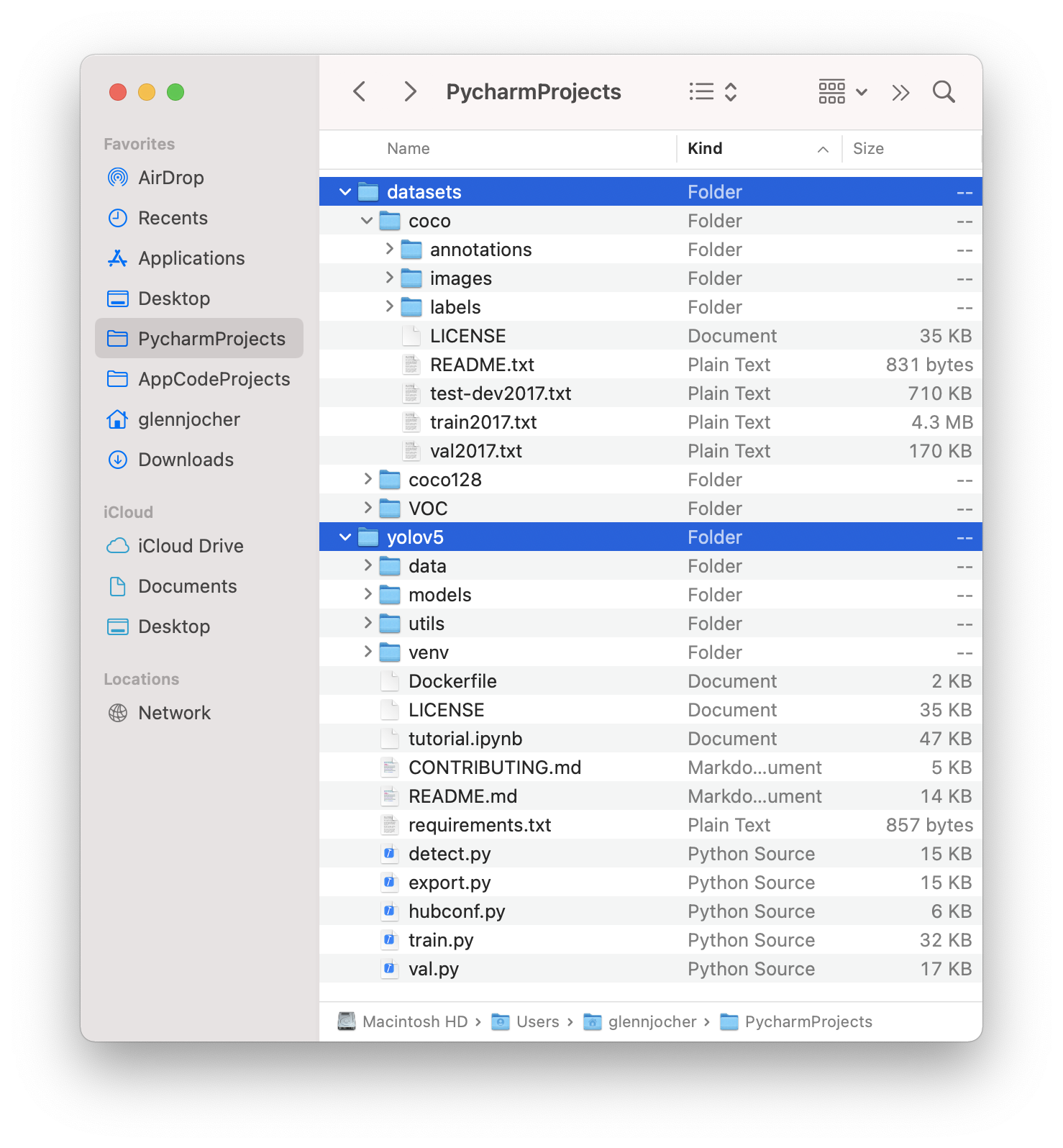
-
-### 2. Select a Model
+## 3. Select a Model
Select a pretrained model to start training from. Here we select [YOLOv5s](https://github.com/ultralytics/yolov5/blob/master/models/yolov5s.yaml), the second-smallest and fastest model available. See our README [table](https://github.com/ultralytics/yolov5#pretrained-checkpoints) for a full comparison of all models.

-### 3. Train
+## 4. Train
Train a YOLOv5s model on COCO128 by specifying dataset, batch-size, image size and either pretrained `--weights yolov5s.pt` (recommended), or randomly initialized `--weights '' --cfg yolov5s.yaml` (not recommended). Pretrained weights are auto-downloaded from the [latest YOLOv5 release](https://github.com/ultralytics/yolov5/releases).
@@ -156,9 +147,9 @@ python train.py --img 640 --epochs 3 --data coco128.yaml --weights yolov5s.pt
All training results are saved to `runs/train/` with incrementing run directories, i.e. `runs/train/exp2`, `runs/train/exp3` etc. For more details see the Training section of our tutorial notebook.
-### 4. Visualize
+## 5. Visualize
-#### Comet Logging and Visualization 🌟 NEW
+### Comet Logging and Visualization 🌟 NEW
[Comet](https://bit.ly/yolov5-readme-comet) is now fully integrated with YOLOv5. Track and visualize model metrics in real time, save your hyperparameters, datasets, and model checkpoints, and visualize your model predictions with [Comet Custom Panels](https://bit.ly/yolov5-colab-comet-panels)! Comet makes sure you never lose track of your work and makes it easy to share results and collaborate across teams of all sizes!
@@ -174,21 +165,21 @@ To learn more about all the supported Comet features for this integration, check
-#### ClearML Logging and Automation 🌟 NEW
+### ClearML Logging and Automation 🌟 NEW
-[ClearML](https://cutt.ly/yolov5-notebook-clearml) is completely integrated into YOLOv5 to track your experimentation, manage dataset versions and even remotely execute training runs. To enable ClearML:
+[ClearML](https://clear.ml/) is completely integrated into YOLOv5 to track your experimentation, manage dataset versions and even remotely execute training runs. To enable ClearML:
- `pip install clearml`
-- run `clearml-init` to connect to a ClearML server (**deploy your own open-source server [here](https://github.com/allegroai/clearml-server)**, or use our free hosted server [here](https://cutt.ly/yolov5-notebook-clearml))
+- run `clearml-init` to connect to a ClearML server
You'll get all the great expected features from an experiment manager: live updates, model upload, experiment comparison etc. but ClearML also tracks uncommitted changes and installed packages for example. Thanks to that ClearML Tasks (which is what we call experiments) are also reproducible on different machines! With only 1 extra line, we can schedule a YOLOv5 training task on a queue to be executed by any number of ClearML Agents (workers).
You can use ClearML Data to version your dataset and then pass it to YOLOv5 simply using its unique ID. This will help you keep track of your data without adding extra hassle. Explore the [ClearML Tutorial](https://docs.ultralytics.com/yolov5/tutorials/clearml_logging_integration) for details!
-
+
-#### Local Logging
+### Local Logging
Training results are automatically logged with [Tensorboard](https://www.tensorflow.org/tensorboard) and [CSV](https://github.com/ultralytics/yolov5/pull/4148) loggers to `runs/train`, with a new experiment directory created for each new training as `runs/train/exp2`, `runs/train/exp3`, etc.
diff --git a/docs/overrides/stylesheets/style.css b/docs/overrides/stylesheets/style.css
index b5840560..7fe3511b 100644
--- a/docs/overrides/stylesheets/style.css
+++ b/docs/overrides/stylesheets/style.css
@@ -47,4 +47,4 @@ div.highlight {
/* Set language dropdown maximum height to screen height */
.md-header .md-select:hover .md-select__inner {
max-height: 75vh;
-}
\ No newline at end of file
+}
diff --git a/examples/YOLOv8-ONNXRuntime/main.py b/examples/YOLOv8-ONNXRuntime/main.py
index e3755a44..e1e83f3d 100644
--- a/examples/YOLOv8-ONNXRuntime/main.py
+++ b/examples/YOLOv8-ONNXRuntime/main.py
@@ -1,3 +1,5 @@
+# Ultralytics YOLO 🚀, AGPL-3.0 license
+
import argparse
import cv2
diff --git a/examples/YOLOv8-OpenCV-ONNX-Python/main.py b/examples/YOLOv8-OpenCV-ONNX-Python/main.py
index c0564d15..8d9c7a50 100644
--- a/examples/YOLOv8-OpenCV-ONNX-Python/main.py
+++ b/examples/YOLOv8-OpenCV-ONNX-Python/main.py
@@ -1,3 +1,5 @@
+# Ultralytics YOLO 🚀, AGPL-3.0 license
+
import argparse
import cv2.dnn
diff --git a/examples/YOLOv8-OpenCV-int8-tflite-Python/main.py b/examples/YOLOv8-OpenCV-int8-tflite-Python/main.py
index 9c23173c..f907d012 100644
--- a/examples/YOLOv8-OpenCV-int8-tflite-Python/main.py
+++ b/examples/YOLOv8-OpenCV-int8-tflite-Python/main.py
@@ -1,3 +1,5 @@
+# Ultralytics YOLO 🚀, AGPL-3.0 license
+
import argparse
import cv2
diff --git a/examples/YOLOv8-Region-Counter/yolov8_region_counter.py b/examples/YOLOv8-Region-Counter/yolov8_region_counter.py
index 82d8b29c..b7e9c3b5 100644
--- a/examples/YOLOv8-Region-Counter/yolov8_region_counter.py
+++ b/examples/YOLOv8-Region-Counter/yolov8_region_counter.py
@@ -1,3 +1,5 @@
+# Ultralytics YOLO 🚀, AGPL-3.0 license
+
import argparse
from collections import defaultdict
from pathlib import Path
diff --git a/examples/YOLOv8-SAHI-Inference-Video/yolov8_sahi.py b/examples/YOLOv8-SAHI-Inference-Video/yolov8_sahi.py
index 37d78114..f2b8274c 100644
--- a/examples/YOLOv8-SAHI-Inference-Video/yolov8_sahi.py
+++ b/examples/YOLOv8-SAHI-Inference-Video/yolov8_sahi.py
@@ -1,3 +1,5 @@
+# Ultralytics YOLO 🚀, AGPL-3.0 license
+
import argparse
from pathlib import Path
diff --git a/examples/YOLOv8-Segmentation-ONNXRuntime-Python/main.py b/examples/YOLOv8-Segmentation-ONNXRuntime-Python/main.py
index 7dd11dc3..141d21b9 100644
--- a/examples/YOLOv8-Segmentation-ONNXRuntime-Python/main.py
+++ b/examples/YOLOv8-Segmentation-ONNXRuntime-Python/main.py
@@ -1,3 +1,5 @@
+# Ultralytics YOLO 🚀, AGPL-3.0 license
+
import argparse
import cv2
diff --git a/tests/test_explorer.py b/tests/test_explorer.py
index 6a0995bc..eed02ae5 100644
--- a/tests/test_explorer.py
+++ b/tests/test_explorer.py
@@ -1,4 +1,4 @@
-import PIL
+# Ultralytics YOLO 🚀, AGPL-3.0 license
from ultralytics import Explorer
from ultralytics.utils import ASSETS
diff --git a/ultralytics/engine/results.py b/ultralytics/engine/results.py
index 82d654ed..5a59ea33 100644
--- a/ultralytics/engine/results.py
+++ b/ultralytics/engine/results.py
@@ -605,7 +605,9 @@ class OBB(BaseTensor):
conf (torch.Tensor | numpy.ndarray): The confidence values of the boxes.
cls (torch.Tensor | numpy.ndarray): The class values of the boxes.
id (torch.Tensor | numpy.ndarray): The track IDs of the boxes (if available).
- xyxyxyxy (torch.Tensor | numpy.ndarray): The boxes in xyxyxyxy format normalized by original image size.
+ xyxyxyxyn (torch.Tensor | numpy.ndarray): The rotated boxes in xyxyxyxy format normalized by original image size.
+ xyxyxyxy (torch.Tensor | numpy.ndarray): The rotated boxes in xyxyxyxy format.
+ xyxy (torch.Tensor | numpy.ndarray): The horizontal boxes in xyxyxyxy format.
data (torch.Tensor): The raw OBB tensor (alias for `boxes`).
Methods:
diff --git a/ultralytics/trackers/basetrack.py b/ultralytics/trackers/basetrack.py
index 1e6dca99..c900cac4 100644
--- a/ultralytics/trackers/basetrack.py
+++ b/ultralytics/trackers/basetrack.py
@@ -1,5 +1,5 @@
# Ultralytics YOLO 🚀, AGPL-3.0 license
-# This module defines the base classes and structures for object tracking in YOLO.
+"""This module defines the base classes and structures for object tracking in YOLO."""
from collections import OrderedDict
diff --git a/ultralytics/trackers/track.py b/ultralytics/trackers/track.py
index c0dd4e45..6dee3921 100644
--- a/ultralytics/trackers/track.py
+++ b/ultralytics/trackers/track.py
@@ -1,3 +1,5 @@
+# Ultralytics YOLO 🚀, AGPL-3.0 license
+
from functools import partial
from pathlib import Path
diff --git a/ultralytics/utils/plotting.py b/ultralytics/utils/plotting.py
index 280a3e28..2ed04f5f 100644
--- a/ultralytics/utils/plotting.py
+++ b/ultralytics/utils/plotting.py
@@ -365,7 +365,6 @@ class Annotator:
self.tf = count_txt_size
tl = self.tf or round(0.002 * (self.im.shape[0] + self.im.shape[1]) / 2) + 1
tf = max(tl - 1, 1)
- gap = int(24 * tl) # gap between in_count and out_count based on line_thickness
# Get text size for in_count and out_count
t_size_in = cv2.getTextSize(str(counts), 0, fontScale=tl / 2, thickness=tf)[0]