mirror of
https://github.com/THU-MIG/yolov10.git
synced 2025-05-23 05:24:22 +08:00
ultralytics 8.0.191
fix yolo checks
for missing packages (#5179)
Co-authored-by: pre-commit-ci[bot] <66853113+pre-commit-ci[bot]@users.noreply.github.com> Co-authored-by: Muhammad Rizwan Munawar <62513924+RizwanMunawar@users.noreply.github.com>
This commit is contained in:
parent
9aaa5d5ed0
commit
525c8b0294
@ -52,22 +52,23 @@ When adding new functions or classes, please include a [Google-style docstring](
|
||||
Example Google-style docstring:
|
||||
|
||||
```python
|
||||
def example_function(arg1: int, arg2: str) -> bool:
|
||||
"""Example function that demonstrates Google-style docstrings.
|
||||
def example_function(arg1: int, arg2: int) -> bool:
|
||||
"""
|
||||
Example function that demonstrates Google-style docstrings.
|
||||
|
||||
Args:
|
||||
arg1 (int): The first argument.
|
||||
arg2 (str): The second argument.
|
||||
arg2 (int): The second argument.
|
||||
|
||||
Returns:
|
||||
bool: True if successful, False otherwise.
|
||||
(bool): True if successful, False otherwise.
|
||||
|
||||
Raises:
|
||||
ValueError: If `arg1` is negative or `arg2` is empty.
|
||||
Examples:
|
||||
>>> result = example_function(1, 2) # returns False
|
||||
"""
|
||||
if arg1 < 0 or not arg2:
|
||||
raise ValueError("Invalid input values")
|
||||
return True
|
||||
if arg1 == arg2:
|
||||
return True
|
||||
return False
|
||||
```
|
||||
|
||||
### GitHub Actions CI Tests
|
||||
|
@ -32,10 +32,10 @@ The output from Ultralytics trackers is consistent with standard object detectio
|
||||
|
||||
## Real-world Applications
|
||||
|
||||
| Transportation | Retail | Aquaculture |
|
||||
|:-----------------------------------:|:-----------------------:|:-----------:|
|
||||
| Transportation | Retail | Aquaculture |
|
||||
|:----------------------------------------------------------------------------------------------------------------------:|:---------------------------------------------------------------------------------------------------------------------:|:-------------------------------------------------------------------------------------------------------------------:|
|
||||
| 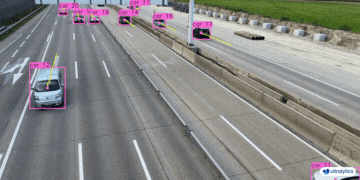 | 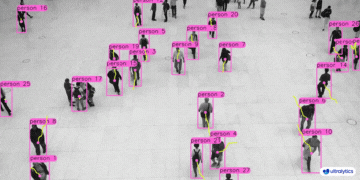 | 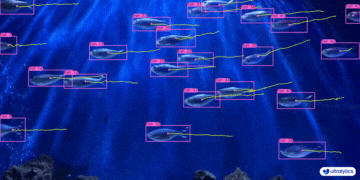 |
|
||||
| Vehicle Tracking | People Tracking | Fish Tracking |
|
||||
| Vehicle Tracking | People Tracking | Fish Tracking |
|
||||
|
||||
## Features at a Glance
|
||||
|
||||
@ -264,7 +264,7 @@ Multithreaded tracking provides the capability to run object tracking on multipl
|
||||
|
||||
In the provided Python script, we make use of Python's `threading` module to run multiple instances of the tracker concurrently. Each thread is responsible for running the tracker on one video file, and all the threads run simultaneously in the background.
|
||||
|
||||
To ensure that each thread receives the correct parameters (the video file and the model to use), we define a function `run_tracker_in_thread` that accepts these parameters and contains the main tracking loop. This function reads the video frame by frame, runs the tracker, and displays the results.
|
||||
To ensure that each thread receives the correct parameters (the video file, the model to use and the file index), we define a function `run_tracker_in_thread` that accepts these parameters and contains the main tracking loop. This function reads the video frame by frame, runs the tracker, and displays the results.
|
||||
|
||||
Two different models are used in this example: `yolov8n.pt` and `yolov8n-seg.pt`, each tracking objects in a different video file. The video files are specified in `video_file1` and `video_file2`.
|
||||
|
||||
@ -276,44 +276,67 @@ Finally, after all threads have completed their task, the windows displaying the
|
||||
|
||||
```python
|
||||
import threading
|
||||
|
||||
import cv2
|
||||
from ultralytics import YOLO
|
||||
|
||||
|
||||
def run_tracker_in_thread(filename, model, file_index):
|
||||
"""
|
||||
Runs a video file or webcam stream concurrently with the YOLOv8 model using threading.
|
||||
|
||||
This function captures video frames from a given file or camera source and utilizes the YOLOv8 model for object
|
||||
tracking. The function runs in its own thread for concurrent processing.
|
||||
|
||||
def run_tracker_in_thread(filename, model):
|
||||
video = cv2.VideoCapture(filename)
|
||||
frames = int(video.get(cv2.CAP_PROP_FRAME_COUNT))
|
||||
for _ in range(frames):
|
||||
ret, frame = video.read()
|
||||
if ret:
|
||||
results = model.track(source=frame, persist=True)
|
||||
res_plotted = results[0].plot()
|
||||
cv2.imshow('p', res_plotted)
|
||||
if cv2.waitKey(1) == ord('q'):
|
||||
break
|
||||
Args:
|
||||
filename (str): The path to the video file or the identifier for the webcam/external camera source.
|
||||
model (obj): The YOLOv8 model object.
|
||||
file_index (int): An index to uniquely identify the file being processed, used for display purposes.
|
||||
|
||||
Note:
|
||||
Press 'q' to quit the video display window.
|
||||
"""
|
||||
video = cv2.VideoCapture(filename) # Read the video file
|
||||
|
||||
while True:
|
||||
ret, frame = video.read() # Read the video frames
|
||||
|
||||
# Exit the loop if no more frames in either video
|
||||
if not ret:
|
||||
break
|
||||
|
||||
# Track objects in frames if available
|
||||
results = model.track(frame, persist=True)
|
||||
res_plotted = results[0].plot()
|
||||
cv2.imshow(f"Tracking_Stream_{file_index}", res_plotted)
|
||||
|
||||
key = cv2.waitKey(1)
|
||||
if key == ord('q'):
|
||||
break
|
||||
|
||||
# Release video sources
|
||||
video.release()
|
||||
|
||||
|
||||
# Load the models
|
||||
model1 = YOLO('yolov8n.pt')
|
||||
model2 = YOLO('yolov8n-seg.pt')
|
||||
|
||||
|
||||
# Define the video files for the trackers
|
||||
video_file1 = 'path/to/video1.mp4'
|
||||
video_file2 = 'path/to/video2.mp4'
|
||||
|
||||
video_file1 = "path/to/video1.mp4" # Path to video file, 0 for webcam
|
||||
video_file2 = 0 # Path to video file, 0 for webcam, 1 for external camera
|
||||
|
||||
# Create the tracker threads
|
||||
tracker_thread1 = threading.Thread(target=run_tracker_in_thread, args=(video_file1, model1), daemon=True)
|
||||
tracker_thread2 = threading.Thread(target=run_tracker_in_thread, args=(video_file2, model2), daemon=True)
|
||||
|
||||
tracker_thread1 = threading.Thread(target=run_tracker_in_thread, args=(video_file1, model1, 1), daemon=True)
|
||||
tracker_thread2 = threading.Thread(target=run_tracker_in_thread, args=(video_file2, model2, 2), daemon=True)
|
||||
|
||||
# Start the tracker threads
|
||||
tracker_thread1.start()
|
||||
tracker_thread2.start()
|
||||
|
||||
|
||||
# Wait for the tracker threads to finish
|
||||
tracker_thread1.join()
|
||||
tracker_thread2.join()
|
||||
|
||||
|
||||
# Clean up and close windows
|
||||
cv2.destroyAllWindows()
|
||||
```
|
||||
|
@ -81,7 +81,7 @@ Train YOLOv8n on the COCO128 dataset for 100 epochs at image size 640. For a ful
|
||||
|
||||
### Dataset format
|
||||
|
||||
YOLO detection dataset format can be found in detail in the [Dataset Guide](../datasets/detect/index.md). To convert your existing dataset from other formats( like COCO etc.) to YOLO format, please use [json2yolo tool](https://github.com/ultralytics/JSON2YOLO) by Ultralytics.
|
||||
YOLO detection dataset format can be found in detail in the [Dataset Guide](../datasets/detect/index.md). To convert your existing dataset from other formats (like COCO etc.) to YOLO format, please use [JSON2YOLO](https://github.com/ultralytics/JSON2YOLO) tool by Ultralytics.
|
||||
|
||||
## Val
|
||||
|
||||
|
@ -84,7 +84,7 @@ Train a YOLOv8-pose model on the COCO128-pose dataset.
|
||||
|
||||
### Dataset format
|
||||
|
||||
YOLO pose dataset format can be found in detail in the [Dataset Guide](../datasets/pose/index.md). To convert your existing dataset from other formats( like COCO etc.) to YOLO format, please use [json2yolo tool](https://github.com/ultralytics/JSON2YOLO) by Ultralytics.
|
||||
YOLO pose dataset format can be found in detail in the [Dataset Guide](../datasets/pose/index.md). To convert your existing dataset from other formats (like COCO etc.) to YOLO format, please use [JSON2YOLO](https://github.com/ultralytics/JSON2YOLO) tool by Ultralytics.
|
||||
|
||||
## Val
|
||||
|
||||
|
@ -81,7 +81,7 @@ Train YOLOv8n-seg on the COCO128-seg dataset for 100 epochs at image size 640. F
|
||||
|
||||
### Dataset format
|
||||
|
||||
YOLO segmentation dataset format can be found in detail in the [Dataset Guide](../datasets/segment/index.md). To convert your existing dataset from other formats( like COCO etc.) to YOLO format, please use [json2yolo tool](https://github.com/ultralytics/JSON2YOLO) by Ultralytics.
|
||||
YOLO segmentation dataset format can be found in detail in the [Dataset Guide](../datasets/segment/index.md). To convert your existing dataset from other formats (like COCO etc.) to YOLO format, please use [JSON2YOLO](https://github.com/ultralytics/JSON2YOLO) tool by Ultralytics.
|
||||
|
||||
## Val
|
||||
|
||||
|
@ -5,10 +5,7 @@ from pathlib import Path
|
||||
|
||||
import pytest
|
||||
|
||||
from ultralytics.utils import ROOT
|
||||
from ultralytics.utils.torch_utils import init_seeds
|
||||
|
||||
TMP = (ROOT / '../tests/tmp').resolve() # temp directory for test files
|
||||
TMP = Path(__file__).resolve().parent / 'tmp' # temp directory for test files
|
||||
|
||||
|
||||
def pytest_addoption(parser):
|
||||
@ -62,6 +59,8 @@ def pytest_sessionstart(session):
|
||||
Args:
|
||||
session (pytest.Session): The pytest session object.
|
||||
"""
|
||||
from ultralytics.utils.torch_utils import init_seeds
|
||||
|
||||
init_seeds()
|
||||
shutil.rmtree(TMP, ignore_errors=True) # delete any existing tests/tmp directory
|
||||
TMP.mkdir(parents=True, exist_ok=True) # create a new empty directory
|
||||
@ -79,10 +78,14 @@ def pytest_terminal_summary(terminalreporter, exitstatus, config):
|
||||
exitstatus (int): The exit status of the test run.
|
||||
config (pytest.config.Config): The pytest config object.
|
||||
"""
|
||||
from ultralytics.utils import WEIGHTS_DIR
|
||||
|
||||
# Remove files
|
||||
for file in ['bus.jpg', 'decelera_landscape_min.mov']:
|
||||
models = [path for x in ['*.onnx', '*.torchscript'] for path in WEIGHTS_DIR.rglob(x)]
|
||||
for file in ['bus.jpg', 'yolov8n.onnx', 'yolov8n.torchscript'] + models:
|
||||
Path(file).unlink(missing_ok=True)
|
||||
|
||||
# Remove directories
|
||||
for directory in [ROOT / '../.pytest_cache', TMP]:
|
||||
models = [path for x in ['*.mlpackage', '*_openvino_model'] for path in WEIGHTS_DIR.rglob(x)]
|
||||
for directory in [TMP.parents[1] / '.pytest_cache', TMP] + models:
|
||||
shutil.rmtree(directory, ignore_errors=True)
|
||||
|
@ -1,16 +1,14 @@
|
||||
# Ultralytics YOLO 🚀, AGPL-3.0 license
|
||||
|
||||
import subprocess
|
||||
from pathlib import Path
|
||||
|
||||
import pytest
|
||||
|
||||
from ultralytics.utils import ASSETS, SETTINGS
|
||||
from ultralytics.utils import ASSETS, WEIGHTS_DIR
|
||||
from ultralytics.utils.checks import cuda_device_count, cuda_is_available
|
||||
|
||||
CUDA_IS_AVAILABLE = cuda_is_available()
|
||||
CUDA_DEVICE_COUNT = cuda_device_count()
|
||||
WEIGHTS_DIR = Path(SETTINGS['weights_dir'])
|
||||
TASK_ARGS = [
|
||||
('detect', 'yolov8n', 'coco8.yaml'),
|
||||
('segment', 'yolov8n-seg', 'coco8-seg.yaml'),
|
||||
|
@ -1,19 +1,16 @@
|
||||
# Ultralytics YOLO 🚀, AGPL-3.0 license
|
||||
import contextlib
|
||||
from pathlib import Path
|
||||
|
||||
import pytest
|
||||
import torch
|
||||
|
||||
from ultralytics import YOLO, download
|
||||
from ultralytics.utils import ASSETS, SETTINGS
|
||||
from ultralytics.utils import ASSETS, DATASETS_DIR, WEIGHTS_DIR
|
||||
from ultralytics.utils.checks import cuda_device_count, cuda_is_available
|
||||
|
||||
CUDA_IS_AVAILABLE = cuda_is_available()
|
||||
CUDA_DEVICE_COUNT = cuda_device_count()
|
||||
|
||||
DATASETS_DIR = Path(SETTINGS['datasets_dir'])
|
||||
WEIGHTS_DIR = Path(SETTINGS['weights_dir'])
|
||||
MODEL = WEIGHTS_DIR / 'path with spaces' / 'yolov8n.pt' # test spaces in path
|
||||
DATA = 'coco8.yaml'
|
||||
BUS = ASSETS / 'bus.jpg'
|
||||
@ -91,7 +88,7 @@ def test_predict_sam():
|
||||
model(ASSETS / 'zidane.jpg', points=[900, 370], labels=[1], device=0)
|
||||
|
||||
# Create SAMPredictor
|
||||
overrides = dict(conf=0.25, task='segment', mode='predict', imgsz=1024, model='mobile_sam.pt')
|
||||
overrides = dict(conf=0.25, task='segment', mode='predict', imgsz=1024, model=WEIGHTS_DIR / 'mobile_sam.pt')
|
||||
predictor = SAMPredictor(overrides=overrides)
|
||||
|
||||
# Set image
|
||||
|
@ -1,18 +1,16 @@
|
||||
# Ultralytics YOLO 🚀, AGPL-3.0 license
|
||||
|
||||
from pathlib import Path
|
||||
|
||||
from ultralytics import YOLO
|
||||
from ultralytics.cfg import get_cfg
|
||||
from ultralytics.engine.exporter import Exporter
|
||||
from ultralytics.models.yolo import classify, detect, segment
|
||||
from ultralytics.utils import ASSETS, DEFAULT_CFG, SETTINGS
|
||||
from ultralytics.utils import ASSETS, DEFAULT_CFG, WEIGHTS_DIR
|
||||
|
||||
CFG_DET = 'yolov8n.yaml'
|
||||
CFG_SEG = 'yolov8n-seg.yaml'
|
||||
CFG_CLS = 'yolov8n-cls.yaml' # or 'squeezenet1_0'
|
||||
CFG = get_cfg(DEFAULT_CFG)
|
||||
MODEL = Path(SETTINGS['weights_dir']) / 'yolov8n'
|
||||
MODEL = WEIGHTS_DIR / 'yolov8n'
|
||||
|
||||
|
||||
def test_func(*args): # noqa
|
||||
|
@ -14,11 +14,11 @@ from torchvision.transforms import ToTensor
|
||||
from ultralytics import RTDETR, YOLO
|
||||
from ultralytics.cfg import TASK2DATA
|
||||
from ultralytics.data.build import load_inference_source
|
||||
from ultralytics.utils import ASSETS, DEFAULT_CFG, LINUX, MACOS, ONLINE, ROOT, SETTINGS, WINDOWS, is_dir_writeable
|
||||
from ultralytics.utils import (ASSETS, DEFAULT_CFG, DEFAULT_CFG_PATH, LINUX, MACOS, ONLINE, ROOT, WEIGHTS_DIR, WINDOWS,
|
||||
is_dir_writeable)
|
||||
from ultralytics.utils.downloads import download
|
||||
from ultralytics.utils.torch_utils import TORCH_1_9
|
||||
|
||||
WEIGHTS_DIR = Path(SETTINGS['weights_dir'])
|
||||
MODEL = WEIGHTS_DIR / 'path with spaces' / 'yolov8n.pt' # test spaces in path
|
||||
CFG = 'yolov8n.yaml'
|
||||
SOURCE = ASSETS / 'bus.jpg'
|
||||
@ -260,12 +260,12 @@ def test_predict_callback_and_setup():
|
||||
|
||||
def test_results():
|
||||
for m in 'yolov8n-pose.pt', 'yolov8n-seg.pt', 'yolov8n.pt', 'yolov8n-cls.pt':
|
||||
results = YOLO(m)([SOURCE, SOURCE], imgsz=160)
|
||||
results = YOLO(WEIGHTS_DIR / m)([SOURCE, SOURCE], imgsz=160)
|
||||
for r in results:
|
||||
r = r.cpu().numpy()
|
||||
r = r.to(device='cpu', dtype=torch.float32)
|
||||
r.save_txt(txt_file='runs/tests/label.txt', save_conf=True)
|
||||
r.save_crop(save_dir='runs/tests/crops/')
|
||||
r.save_txt(txt_file=TMP / 'runs/tests/label.txt', save_conf=True)
|
||||
r.save_crop(save_dir=TMP / 'runs/tests/crops/')
|
||||
r.tojson(normalize=True)
|
||||
r.plot(pil=True)
|
||||
r.plot(conf=True, boxes=True)
|
||||
@ -299,14 +299,17 @@ def test_data_converter():
|
||||
|
||||
file = 'instances_val2017.json'
|
||||
download(f'https://github.com/ultralytics/yolov5/releases/download/v1.0/{file}', dir=TMP)
|
||||
convert_coco(labels_dir=TMP, use_segments=True, use_keypoints=False, cls91to80=True)
|
||||
convert_coco(labels_dir=TMP, save_dir=TMP / 'yolo_labels', use_segments=True, use_keypoints=False, cls91to80=True)
|
||||
coco80_to_coco91_class()
|
||||
|
||||
|
||||
def test_data_annotator():
|
||||
from ultralytics.data.annotator import auto_annotate
|
||||
|
||||
auto_annotate(ASSETS, det_model='yolov8n.pt', sam_model='mobile_sam.pt', output_dir=TMP / 'auto_annotate_labels')
|
||||
auto_annotate(ASSETS,
|
||||
det_model=WEIGHTS_DIR / 'yolov8n.pt',
|
||||
sam_model=WEIGHTS_DIR / 'mobile_sam.pt',
|
||||
output_dir=TMP / 'auto_annotate_labels')
|
||||
|
||||
|
||||
def test_events():
|
||||
@ -326,6 +329,7 @@ def test_cfg_init():
|
||||
with contextlib.suppress(SyntaxError):
|
||||
check_dict_alignment({'a': 1}, {'b': 2})
|
||||
copy_default_cfg()
|
||||
(Path.cwd() / DEFAULT_CFG_PATH.name.replace('.yaml', '_copy.yaml')).unlink(missing_ok=False)
|
||||
[smart_value(x) for x in ['none', 'true', 'false']]
|
||||
|
||||
|
||||
|
@ -1,6 +1,6 @@
|
||||
# Ultralytics YOLO 🚀, AGPL-3.0 license
|
||||
|
||||
__version__ = '8.0.190'
|
||||
__version__ = '8.0.191'
|
||||
|
||||
from ultralytics.models import RTDETR, SAM, YOLO
|
||||
from ultralytics.models.fastsam import FastSAM
|
||||
|
@ -7,9 +7,9 @@ from pathlib import Path
|
||||
from types import SimpleNamespace
|
||||
from typing import Dict, List, Union
|
||||
|
||||
from ultralytics.utils import (ASSETS, DEFAULT_CFG, DEFAULT_CFG_DICT, DEFAULT_CFG_PATH, LOGGER, RANK, SETTINGS,
|
||||
SETTINGS_YAML, IterableSimpleNamespace, __version__, checks, colorstr, deprecation_warn,
|
||||
yaml_load, yaml_print)
|
||||
from ultralytics.utils import (ASSETS, DEFAULT_CFG, DEFAULT_CFG_DICT, DEFAULT_CFG_PATH, LOGGER, RANK, ROOT, SETTINGS,
|
||||
SETTINGS_YAML, TESTS_RUNNING, IterableSimpleNamespace, __version__, checks, colorstr,
|
||||
deprecation_warn, yaml_load, yaml_print)
|
||||
|
||||
# Define valid tasks and modes
|
||||
MODES = 'train', 'val', 'predict', 'export', 'track', 'benchmark'
|
||||
@ -153,7 +153,8 @@ def get_save_dir(args, name=None):
|
||||
else:
|
||||
from ultralytics.utils.files import increment_path
|
||||
|
||||
project = args.project or Path(SETTINGS['runs_dir']) / args.task
|
||||
project = args.project or (ROOT /
|
||||
'../tests/tmp/runs' if TESTS_RUNNING else Path(SETTINGS['runs_dir'])) / args.task
|
||||
name = name or args.name or f'{args.mode}'
|
||||
save_dir = increment_path(Path(project) / name, exist_ok=args.exist_ok if RANK in (-1, 0) else True)
|
||||
|
||||
|
@ -46,11 +46,16 @@ def coco80_to_coco91_class(): #
|
||||
64, 65, 67, 70, 72, 73, 74, 75, 76, 77, 78, 79, 80, 81, 82, 84, 85, 86, 87, 88, 89, 90]
|
||||
|
||||
|
||||
def convert_coco(labels_dir='../coco/annotations/', use_segments=False, use_keypoints=False, cls91to80=True):
|
||||
def convert_coco(labels_dir='../coco/annotations/',
|
||||
save_dir='.',
|
||||
use_segments=False,
|
||||
use_keypoints=False,
|
||||
cls91to80=True):
|
||||
"""Converts COCO dataset annotations to a format suitable for training YOLOv5 models.
|
||||
|
||||
Args:
|
||||
labels_dir (str, optional): Path to directory containing COCO dataset annotation files.
|
||||
save_dir (str, optional): Path to directory to save results to.
|
||||
use_segments (bool, optional): Whether to include segmentation masks in the output.
|
||||
use_keypoints (bool, optional): Whether to include keypoint annotations in the output.
|
||||
cls91to80 (bool, optional): Whether to map 91 COCO class IDs to the corresponding 80 COCO class IDs.
|
||||
@ -67,7 +72,7 @@ def convert_coco(labels_dir='../coco/annotations/', use_segments=False, use_keyp
|
||||
"""
|
||||
|
||||
# Create dataset directory
|
||||
save_dir = Path('yolo_labels')
|
||||
save_dir = Path(save_dir)
|
||||
if save_dir.exists():
|
||||
shutil.rmtree(save_dir) # delete dir
|
||||
for p in save_dir / 'labels', save_dir / 'images':
|
||||
|
@ -148,11 +148,12 @@ sam_model_map = {
|
||||
def build_sam(ckpt='sam_b.pt'):
|
||||
"""Build a SAM model specified by ckpt."""
|
||||
model_builder = None
|
||||
ckpt = str(ckpt) # to allow Path ckpt types
|
||||
for k in sam_model_map.keys():
|
||||
if ckpt.endswith(k):
|
||||
model_builder = sam_model_map.get(k)
|
||||
|
||||
if not model_builder:
|
||||
raise FileNotFoundError(f'{ckpt} is not a supported sam model. Available models are: \n {sam_model_map.keys()}')
|
||||
raise FileNotFoundError(f'{ckpt} is not a supported SAM model. Available models are: \n {sam_model_map.keys()}')
|
||||
|
||||
return model_builder(ckpt)
|
||||
|
@ -917,6 +917,7 @@ def url2file(url):
|
||||
PREFIX = colorstr('Ultralytics: ')
|
||||
SETTINGS = SettingsManager() # initialize settings
|
||||
DATASETS_DIR = Path(SETTINGS['datasets_dir']) # global datasets directory
|
||||
WEIGHTS_DIR = Path(SETTINGS['weights_dir'])
|
||||
ENVIRONMENT = 'Colab' if is_colab() else 'Kaggle' if is_kaggle() else 'Jupyter' if is_jupyter() else \
|
||||
'Docker' if is_docker() else platform.system()
|
||||
TESTS_RUNNING = is_pytest_running() or is_github_actions_ci()
|
||||
|
@ -36,13 +36,13 @@ import torch.cuda
|
||||
from ultralytics import YOLO
|
||||
from ultralytics.cfg import TASK2DATA, TASK2METRIC
|
||||
from ultralytics.engine.exporter import export_formats
|
||||
from ultralytics.utils import ASSETS, LINUX, LOGGER, MACOS, SETTINGS, TQDM
|
||||
from ultralytics.utils import ASSETS, LINUX, LOGGER, MACOS, TQDM, WEIGHTS_DIR
|
||||
from ultralytics.utils.checks import check_requirements, check_yolo
|
||||
from ultralytics.utils.files import file_size
|
||||
from ultralytics.utils.torch_utils import select_device
|
||||
|
||||
|
||||
def benchmark(model=Path(SETTINGS['weights_dir']) / 'yolov8n.pt',
|
||||
def benchmark(model=WEIGHTS_DIR / 'yolov8n.pt',
|
||||
data=None,
|
||||
imgsz=160,
|
||||
half=False,
|
||||
|
@ -516,8 +516,12 @@ def collect_system_info():
|
||||
f"{'CUDA':<20}{torch.version.cuda if torch and torch.cuda.is_available() else None}\n")
|
||||
|
||||
for r in parse_requirements(package='ultralytics'):
|
||||
current = metadata.version(r.name)
|
||||
is_met = '✅ ' if check_version(current, str(r.specifier)) else '❌ '
|
||||
try:
|
||||
current = metadata.version(r.name)
|
||||
is_met = '✅ ' if check_version(current, str(r.specifier), hard=True) else '❌ '
|
||||
except metadata.PackageNotFoundError:
|
||||
current = '(not installed)'
|
||||
is_met = '❌ '
|
||||
LOGGER.info(f'{r.name:<20}{is_met}{current}{r.specifier}')
|
||||
|
||||
|
||||
|
Loading…
x
Reference in New Issue
Block a user