diff --git a/README.md b/README.md
index bb3b596d..5d8eaf40 100644
--- a/README.md
+++ b/README.md
@@ -1,295 +1,85 @@
-
-
-
-
-
+# [YOLOv10: Real-Time End-to-End Object Detection]()
-[中文](https://docs.ultralytics.com/zh/) | [한국어](https://docs.ultralytics.com/ko/) | [日本語](https://docs.ultralytics.com/ja/) | [Русский](https://docs.ultralytics.com/ru/) | [Deutsch](https://docs.ultralytics.com/de/) | [Français](https://docs.ultralytics.com/fr/) | [Español](https://docs.ultralytics.com/es/) | [Português](https://docs.ultralytics.com/pt/) | [हिन्दी](https://docs.ultralytics.com/hi/) | [العربية](https://docs.ultralytics.com/ar/)
-
-
+Official PyTorch implementation of **YOLOv10**.
-[Ultralytics](https://ultralytics.com) [YOLOv8](https://github.com/ultralytics/ultralytics) is a cutting-edge, state-of-the-art (SOTA) model that builds upon the success of previous YOLO versions and introduces new features and improvements to further boost performance and flexibility. YOLOv8 is designed to be fast, accurate, and easy to use, making it an excellent choice for a wide range of object detection and tracking, instance segmentation, image classification and pose estimation tasks.
+
+
+
+ Comparisons with others in terms of latency-accuracy (left) and size-accuracy (right) trade-offs.
+
-We hope that the resources here will help you get the most out of YOLOv8. Please browse the YOLOv8
Docs for details, raise an issue on
GitHub for support, and join our
Discord community for questions and discussions!
+[YOLOv10: Real-Time End-to-End Object Detection]().\
+Ao Wang, Hui Chen, Lihao Liu, Kai Chen, Zijia Lin, Jungong Han, and Guiguang Ding\
+[[`arXiv`]()]
-To request an Enterprise License please complete the form at [Ultralytics Licensing](https://ultralytics.com/license).
+
+
+ Abstract
+
+Over the past years, YOLOs have emerged as the predominant paradigm in the field of real-time object detection owing to their effective balance between computational cost and detection performance. Researchers have explored the architectural designs, optimization objectives, data augmentation strategies, and others for YOLOs, achieving notable progress. However, the reliance on the non-maximum suppression (NMS) for post-processing hampers the end-to-end deployment of YOLOs and adversely impacts the inference latency. Besides, the design of various components in YOLOs lacks the comprehensive and thorough inspection, resulting in noticeable computational redundancy and limiting the model's capability. It renders the suboptimal efficiency, along with considerable potential for performance improvements. In this work, we aim to further advance the performance-efficiency boundary of YOLOs from both the post-processing and the model architecture. To this end, we first present the consistent dual assignments for NMS-free training of YOLOs, which brings the competitive performance and low inference latency simultaneously. Moreover, we introduce the holistic efficiency-accuracy driven model design strategy for YOLOs. We comprehensively optimize various components of YOLOs from both the efficiency and accuracy perspectives, which greatly reduces the computational overhead and enhances the capability. The outcome of our effort is a new generation of YOLO series for real-time end-to-end object detection, dubbed YOLOv10. Extensive experiments show that YOLOv10 achieves the state-of-the-art performance and efficiency across various model scales. For example, our YOLOv10-S is 1.8$\times$ faster than RT-DETR-R18 under the similar AP on COCO, meanwhile enjoying 2.8$\times$ smaller number of parameters and FLOPs. Compared with YOLOv9-C, YOLOv10-B has 46\% less latency and 25\% fewer parameters for the same performance.
+
-
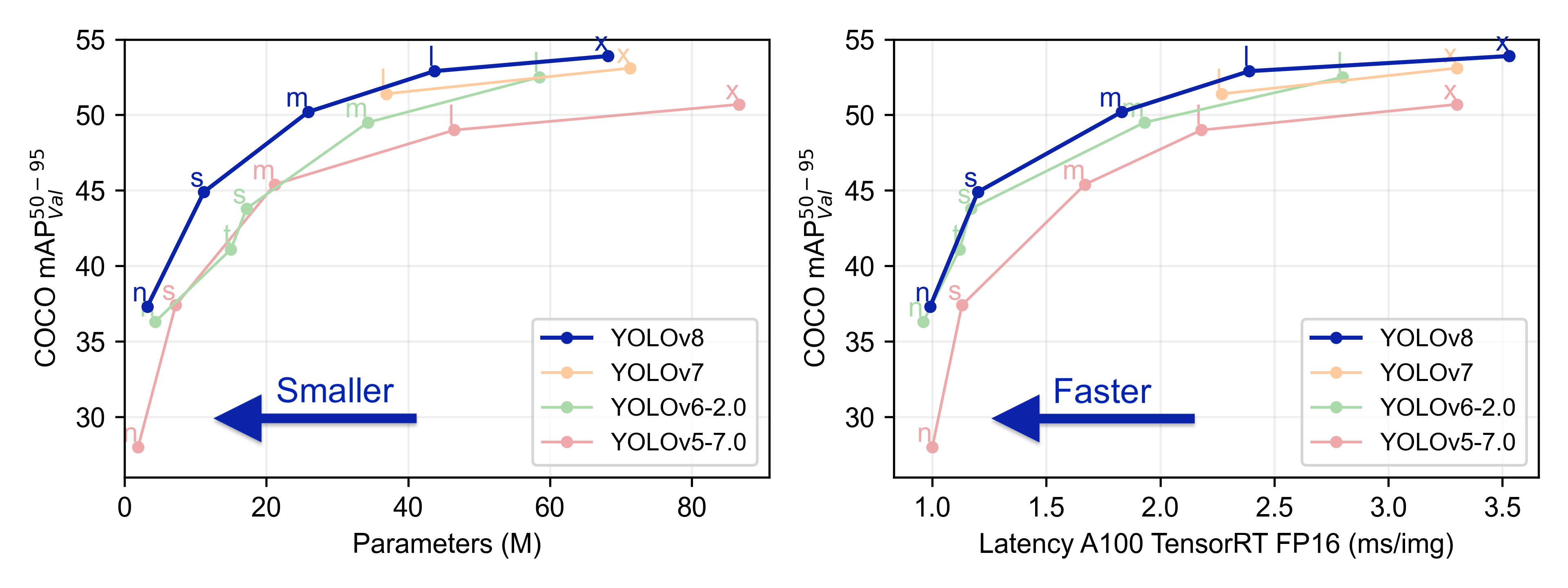
+## Performance
+COCO
+| Model | Test Size | #Params | FLOPs | AP
val | Latency |
+|:---------------|:----:|:---:|:--:|:--:|:--:|
+| [YOLOv10-N](https://github.com/jameslahm/yolov10/releases/download/v1.0/yolov10n.pt) | 640 | 2.3M | 6.7G | 38.5% | 1.84ms |
+| [YOLOv10-S](https://github.com/jameslahm/yolov10/releases/download/v1.0/yolov10s.pt) | 640 | 7.2M | 21.6G | 46.3% | 2.49ms |
+| [YOLOv10-M](https://github.com/jameslahm/yolov10/releases/download/v1.0/yolov10m.pt) | 640 | 15.4M | 59.1G | 51.1% | 4.74ms |
+| [YOLOv10-B](https://github.com/jameslahm/yolov10/releases/download/v1.0/yolov10b.pt) | 640 | 19.1M | 92.0G | 52.5% | 5.74ms |
+| [YOLOv10-L](https://github.com/jameslahm/yolov10/releases/download/v1.0/yolov10l.pt) | 640 | 24.4M | 120.3G | 53.2% | 7.28ms |
+| [YOLOv10-X](https://github.com/jameslahm/yolov10/releases/download/v1.0/yolov10x.pt) | 640 | 29.5M | 160.4G | 54.4% | 10.70ms |
-
-

-

-

-

-

-

-

-

-

-

-

-

-

-
-
-
-##
-
-
-
-
-
-[中文](https://docs.ultralytics.com/zh/) | [한국어](https://docs.ultralytics.com/ko/) | [日本語](https://docs.ultralytics.com/ja/) | [Русский](https://docs.ultralytics.com/ru/) | [Deutsch](https://docs.ultralytics.com/de/) | [Français](https://docs.ultralytics.com/fr/) | [Español](https://docs.ultralytics.com/es/) | [Português](https://docs.ultralytics.com/pt/) | [हिन्दी](https://docs.ultralytics.com/hi/) | [العربية](https://docs.ultralytics.com/ar/)
-
-
-
-
-[Ultralytics](https://ultralytics.com) [YOLOv8](https://github.com/ultralytics/ultralytics) 是一款前沿、最先进(SOTA)的模型,基于先前 YOLO 版本的成功,引入了新功能和改进,进一步提升性能和灵活性。YOLOv8 设计快速、准确且易于使用,使其成为各种物体检测与跟踪、实例分割、图像分类和姿态估计任务的绝佳选择。
-
-我们希望这里的资源能帮助您充分利用 YOLOv8。请浏览 YOLOv8
文档 了解详细信息,在
GitHub 上提交问题以获得支持,并加入我们的
Discord 社区进行问题和讨论!
-
-如需申请企业许可,请在 [Ultralytics Licensing](https://ultralytics.com/license) 处填写表格
-
-
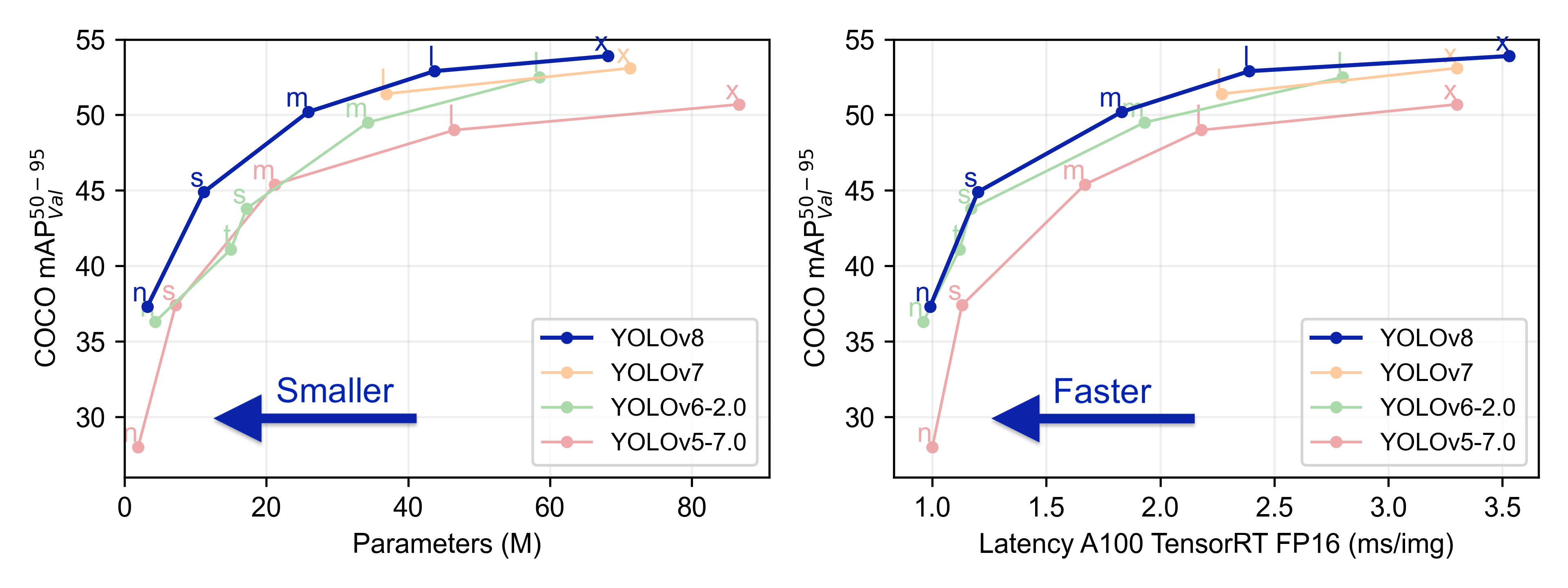
-
-
-

-

-

-

-

-

-

-

-

-

-

-

-

-
-
-
-以下是提供的内容的中文翻译:
-
-##