mirror of
https://github.com/THU-MIG/yolov10.git
synced 2025-05-24 06:14:55 +08:00
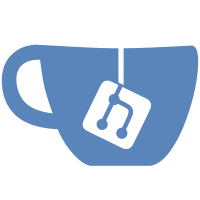
Signed-off-by: dependabot[bot] <support@github.com> Co-authored-by: Glenn Jocher <glenn.jocher@ultralytics.com> Co-authored-by: pre-commit-ci[bot] <66853113+pre-commit-ci[bot]@users.noreply.github.com> Co-authored-by: Mert Can Demir <validatedev@gmail.com> Co-authored-by: Laughing <61612323+Laughing-q@users.noreply.github.com> Co-authored-by: Fabian Greavu <fabiangreavu@gmail.com> Co-authored-by: Yonghye Kwon <developer.0hye@gmail.com> Co-authored-by: Eric Pedley <ericpedley@gmail.com> Co-authored-by: JustasBart <40023722+JustasBart@users.noreply.github.com> Co-authored-by: dependabot[bot] <49699333+dependabot[bot]@users.noreply.github.com> Co-authored-by: Aarni Koskela <akx@iki.fi> Co-authored-by: Sergio Sanchez <sergio.ssm.97@gmail.com> Co-authored-by: Bogdan Gheorghe <112427971+bogdan-galileo@users.noreply.github.com> Co-authored-by: Jaap van de Loosdrecht <jaap@vdlmv.nl> Co-authored-by: Noobtoss <96134731+Noobtoss@users.noreply.github.com> Co-authored-by: nerdyespresso <106761627+nerdyespresso@users.noreply.github.com> Co-authored-by: Farid Inawan <frdteknikelektro@gmail.com> Co-authored-by: Laughing-q <1185102784@qq.com> Co-authored-by: Alexander Duda <Alexander.Duda@me.com> Co-authored-by: Mehran Ghandehari <mehran.maps@gmail.com> Co-authored-by: Snyk bot <snyk-bot@snyk.io> Co-authored-by: majid nasiri <majnasai@gmail.com>
71 lines
2.8 KiB
Python
71 lines
2.8 KiB
Python
# Ultralytics YOLO 🚀, GPL-3.0 license
|
|
|
|
import torch
|
|
import torch.nn as nn
|
|
import torch.nn.functional as F
|
|
|
|
from .metrics import bbox_iou
|
|
from .tal import bbox2dist
|
|
|
|
|
|
class VarifocalLoss(nn.Module):
|
|
# Varifocal loss by Zhang et al. https://arxiv.org/abs/2008.13367
|
|
def __init__(self):
|
|
super().__init__()
|
|
|
|
def forward(self, pred_score, gt_score, label, alpha=0.75, gamma=2.0):
|
|
weight = alpha * pred_score.sigmoid().pow(gamma) * (1 - label) + gt_score * label
|
|
with torch.cuda.amp.autocast(enabled=False):
|
|
loss = (F.binary_cross_entropy_with_logits(pred_score.float(), gt_score.float(), reduction='none') *
|
|
weight).sum()
|
|
return loss
|
|
|
|
|
|
class BboxLoss(nn.Module):
|
|
|
|
def __init__(self, reg_max, use_dfl=False):
|
|
super().__init__()
|
|
self.reg_max = reg_max
|
|
self.use_dfl = use_dfl
|
|
|
|
def forward(self, pred_dist, pred_bboxes, anchor_points, target_bboxes, target_scores, target_scores_sum, fg_mask):
|
|
# IoU loss
|
|
weight = torch.masked_select(target_scores.sum(-1), fg_mask).unsqueeze(-1)
|
|
iou = bbox_iou(pred_bboxes[fg_mask], target_bboxes[fg_mask], xywh=False, CIoU=True)
|
|
loss_iou = ((1.0 - iou) * weight).sum() / target_scores_sum
|
|
|
|
# DFL loss
|
|
if self.use_dfl:
|
|
target_ltrb = bbox2dist(anchor_points, target_bboxes, self.reg_max)
|
|
loss_dfl = self._df_loss(pred_dist[fg_mask].view(-1, self.reg_max + 1), target_ltrb[fg_mask]) * weight
|
|
loss_dfl = loss_dfl.sum() / target_scores_sum
|
|
else:
|
|
loss_dfl = torch.tensor(0.0).to(pred_dist.device)
|
|
|
|
return loss_iou, loss_dfl
|
|
|
|
@staticmethod
|
|
def _df_loss(pred_dist, target):
|
|
# Return sum of left and right DFL losses
|
|
# Distribution Focal Loss (DFL) proposed in Generalized Focal Loss https://ieeexplore.ieee.org/document/9792391
|
|
tl = target.long() # target left
|
|
tr = tl + 1 # target right
|
|
wl = tr - target # weight left
|
|
wr = 1 - wl # weight right
|
|
return (F.cross_entropy(pred_dist, tl.view(-1), reduction='none').view(tl.shape) * wl +
|
|
F.cross_entropy(pred_dist, tr.view(-1), reduction='none').view(tl.shape) * wr).mean(-1, keepdim=True)
|
|
|
|
|
|
class KeypointLoss(nn.Module):
|
|
|
|
def __init__(self, sigmas) -> None:
|
|
super().__init__()
|
|
self.sigmas = sigmas
|
|
|
|
def forward(self, pred_kpts, gt_kpts, kpt_mask, area):
|
|
d = (pred_kpts[..., 0] - gt_kpts[..., 0]) ** 2 + (pred_kpts[..., 1] - gt_kpts[..., 1]) ** 2
|
|
kpt_loss_factor = (torch.sum(kpt_mask != 0) + torch.sum(kpt_mask == 0)) / (torch.sum(kpt_mask != 0) + 1e-9)
|
|
# e = d / (2 * (area * self.sigmas) ** 2 + 1e-9) # from formula
|
|
e = d / (2 * self.sigmas) ** 2 / (area + 1e-9) / 2 # from cocoeval
|
|
return kpt_loss_factor * ((1 - torch.exp(-e)) * kpt_mask).mean()
|