mirror of
https://github.com/THU-MIG/yolov10.git
synced 2025-05-23 13:34:23 +08:00
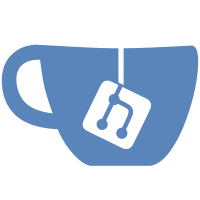
Signed-off-by: Glenn Jocher <glenn.jocher@ultralytics.com> Co-authored-by: pre-commit-ci[bot] <66853113+pre-commit-ci[bot]@users.noreply.github.com>
77 lines
3.8 KiB
Markdown
77 lines
3.8 KiB
Markdown
---
|
||
comments: true
|
||
description: YOLOv8モデルをONNX, TensorRT, CoreMLなどの様々なフォーマットへエキスポートする手順についてのガイドです。今すぐ展開を探求してください!
|
||
keywords: YOLO, YOLOv8, Ultralytics, モデルエキスポート, ONNX, TensorRT, CoreML, TensorFlow SavedModel, OpenVINO, PyTorch, モデルをエキスポート
|
||
---
|
||
|
||
# Ultralytics YOLO でのモデルエキスポート
|
||
|
||
<img width="1024" src="https://github.com/ultralytics/assets/raw/main/yolov8/banner-integrations.png" alt="Ultralytics YOLO エコシステムと統合">
|
||
|
||
## はじめに
|
||
|
||
モデルのトレーニング終了後の最終目標は、実世界のアプリケーションに導入することです。Ultralytics YOLOv8のエキスポートモードは、トレーニング済みモデルを異なるフォーマットにエキスポートして、様々なプラットフォームやデバイスで展開可能にするための多様なオプションを提供します。この包括的なガイドは、モデルエキスポートのニュアンスをわかりやすく解説し、最大の互換性とパフォーマンスを達成する方法をご紹介します。
|
||
|
||
<p align="center">
|
||
<br>
|
||
<iframe width="720" height="405" src="https://www.youtube.com/embed/WbomGeoOT_k?si=aGmuyooWftA0ue9X"
|
||
title="YouTube video player" frameborder="0"
|
||
allow="accelerometer; autoplay; clipboard-write; encrypted-media; gyroscope; picture-in-picture; web-share"
|
||
allowfullscreen>
|
||
</iframe>
|
||
<br>
|
||
<strong>視聴:</strong> カスタムトレーニングしたUltralytics YOLOv8モデルをエキスポートして、ウェブカムでリアルタイム推論を実行する方法。
|
||
</p>
|
||
|
||
## YOLOv8のエキスポートモードを選ぶ理由は?
|
||
|
||
- **汎用性:** ONNX, TensorRT, CoreMLなど複数のフォーマットへエキスポート。
|
||
- **パフォーマンス:** TensorRTで最大5倍のGPU高速化、ONNXまたはOpenVINOで3倍のCPU高速化を実現。
|
||
- **互換性:** 様々なハードウェアおよびソフトウェア環境でユニバーサルにモデルを展開。
|
||
- **使いやすさ:** シンプルなCLIおよびPython APIで簡単かつ迅速なモデルエキスポート。
|
||
|
||
### エキスポートモードの主要機能
|
||
|
||
いくつかの注目すべき機能は以下の通りです:
|
||
|
||
- **ワンクリックエキスポート:** 異なるフォーマットへのシンプルなコマンド。
|
||
- **バッチエキスポート:** バッチ推論対応モデルをエキスポート。
|
||
- **最適化推論:** より高速な推論のために最適化されたエキスポートモデル。
|
||
- **チュートリアル動画:** スムーズなエキスポート体験のための詳細なガイドとチュートリアル。
|
||
|
||
!!! Tip "ヒント"
|
||
|
||
* ONNXまたはOpenVINOへのエキスポートで最大3倍のCPU速度アップ。
|
||
* TensorRTへのエキスポートで最大5倍のGPU速度アップ。
|
||
|
||
## 使用例
|
||
|
||
YOLOv8nモデルをONNXやTensorRTなどの異なるフォーマットにエキスポートします。エキスポート引数のフルリストについては、以下のArgumentsセクションをご覧ください。
|
||
|
||
!!! Example "例"
|
||
|
||
=== "Python"
|
||
|
||
```python
|
||
from ultralytics import YOLO
|
||
|
||
# Load a model
|
||
model = YOLO('yolov8n.pt') # 公式モデルを読み込む
|
||
model = YOLO('path/to/best.pt') # カスタムトレーニングモデルを読み込む
|
||
|
||
# モデルをエキスポート
|
||
model.export(format='onnx')
|
||
```
|
||
=== "CLI"
|
||
|
||
```bash
|
||
yolo export model=yolov8n.pt format=onnx # 公式モデルをエキスポート
|
||
yolo export model=path/to/best.pt format=onnx # カスタムトレーニングモデルをエキスポート
|
||
```
|
||
|
||
## 引数
|
||
|
||
YOLOモデルのエキスポート設定
|
||
|
||
[...content truncated for length...]
|